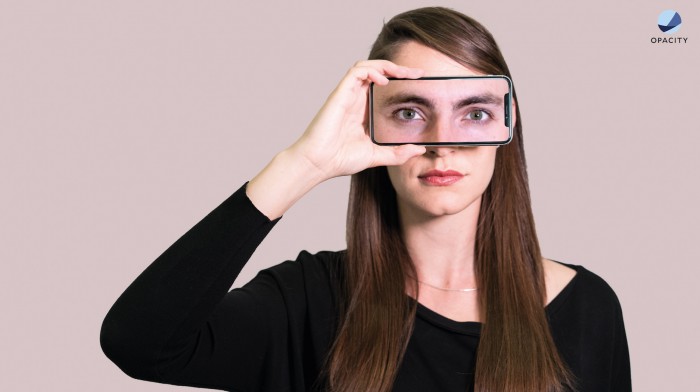
The Science Fiction industry has long-fed the human imagination with images of robots surpassing the human race. While the hype of artificial intelligence only grows with data scientists insisting on its imminent reality. Fortunately for us, we are not yet competing with humanoid robots for our survival, but we are competing with them on the professional level.
Machine learning models make it possible for people to feed big data to algorithms which predict outcomes based on the patterns in that data. And, considering machines are able to consume and analyse more data, more efficiently than the human-eye and without human biases, the results tend to be more accurate and save time.
However, when we start to blindly give machines decision-making power based on their precision and objectiveness, we may, unintentionally, be perpetuating prejudices ingrained in the data. This is because the predictions made by algorithms are entirely based on the data provided by people. If the data is biased, the model will be too. This is an ever-more problematic situation as business processes become further automated.
Earlier this year, a study by MIT and Microsoft showed that facial recognition technology from IBM, Microsoft and Megvii was around 99 per cent accurate at identifying a white male face, but its accuracy dropped to 35 per cent when presented with the face of a woman of colour. This shows that the data used to train the software was skewed towards recognising the features of white men.
The bias becomes even scarier when the predictions are used as evidence for a person’s character. Many companies are looking to replace time-consuming tasks with automated models, such as filtering through CVs before making a hiring decision. In these cases, the company would use their previous employment and promotion information to build the machine learning model. The algorithm would then look for patterns on what qualities make a successful hire. However, if a company has historically hired and promoted more white males due to a racial and gender bias, the model will use this information in order to score future candidates.
In this way, human sexism, racism and prejudice can easily be adopted by these “innovative” technologies if we are not aware of the bias in the data.
The global pivot to advanced data science solutions has allowed such automations to go unnoticed. Big data analysis needs to be treated like a scientific experiment; clearly defining the confounds and biases and trying to mitigate them.
Data science in the academic world is peer-reviewed which provides an extra layer of accountability on published papers. Corporations, on the other hand, are not held accountable such as in the scientific community.
One way to encourage bias-checking in data science is by increasing user awareness of automated systems. Mikhail Wertheim Aymès is a design student from the Royal College of Art who is trying to accomplish this with his service, OPACITY. According to Aymès, “The OPACITY is an independent service that consults with companies in the development phase, using design thinking as [a] tool to help AI developers plan with user ethics at its core.”
The service provides companies with a digital AI certification “similar to a fairtrade symbol.” The public, as consumers or professionals, can then decide how to interact with the company based on the trustmark awarded by OPACITY. In this way, companies will be incentivised to check their automated models for possible biases.
As we try to mitigate the prejudices of the past in our society, it is important to ensure that we also do so in the technology we build. The future of our interaction with AI is already riddled with uncertainty, but one thing is for sure: AI will reflect who we are in many ways. The onus stands with us today to ensure our technologies do not inherit our poorest human qualities, and tackling embedded prejudices should be at the forefront of this initiative.
More on technology and design:
Eliminating the enormous energy cost of mining cryptocurrencies
How the repeal of net neutrality will affect innovation
FrailTea analyses kettle grip data to monitor the health of the elderly