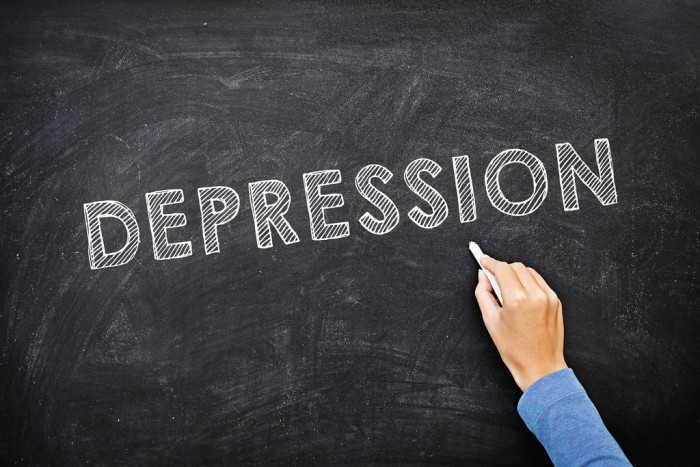
Using machine learning and neural network models to detect depression or other cognitive impairment is not a novel concept. Machine-learning models, for instance, have been developed that can detect words and intonations of speech that may indicate depression.
But these models tend to predict that a person is depressed or not, based on the person’s specific answers to specific questions. Natural, everyday interactions don’t happen this way.
“The first hints we have that a person is happy, excited, sad, or has some serious cognitive condition, such as depression, is through their speech,” says author Tuka Alhanai, a researcher in the Computer Science and Artificial Intelligence Laboratory (CSAIL) at MIT.
To use machine-learning to detect depression in a scalable way, you need to remove the constraints, namely the clinical question and answer session. “You want to deploy it in any regular conversation and have the model pick up, from the natural interaction, the state of the individual,” adds Alhanai.
To do this Alhanai and the team at MIT have come up with a new neural network model that can be used on raw text and audio data from interviews to discover speech patterns indicative of depression.
Given a new subject, it can accurately predict if the individual is depressed, without needing any other information about the questions and answers.
While the technology is more efficient on text than order, the study’s results show promise. “We call it ‘context-free,’ because you’re not putting any constraints into the types of questions you’re looking for and the type of responses to those questions,” Alhanai says.
This means that the method can be used for identifying mental distress in casual conversations in clinical offices, adds co-author James Glass, a senior research scientist in CSAIL. “Every patient will talk differently, and if the model sees changes maybe it will be a flag to the doctors,” he says. “This is a step forward in seeing if we can do something assistive to help clinicians.”
The team hopes that, among other uses, the innovation will go on to power mobile apps that monitor a user’s text and voice for mental distress and send alerts. This could be especially useful for those who can’t get to a clinician for an initial diagnosis, due to distance, cost, or a lack of awareness that something may be wrong.
Rob Matheson from the MIT News office has more on the study behind the technology. Keep tabs on Design Indaba for more innovations in mental health as we observe World Suicide Prevention Day, an awareness day observed on 10 September every year, in order to provide worldwide commitment and action to prevent suicides.
Read More:
Falling: Representing depression through art
How can photography help us understand depression?
Hyphen Labs is using VR to create safe spaces for women of colour
photo credit: mikecohen1872 depression via photopin (license)